Learn to use Hybrid Search to increase the relevancy of similarity search results by combining keyword-based sparse vector search with the semantic understanding of dense vector search. With KDB.AI, users have the flexibility to perform either a dense or sparse vector search independently or execute a hybrid search to return re-ranked results based on both approaches. Hybrid search can also combine sparse vector search with KDB.AI temporal search methods such as Transformed and Non-Transformed Temporal Similarity Search.
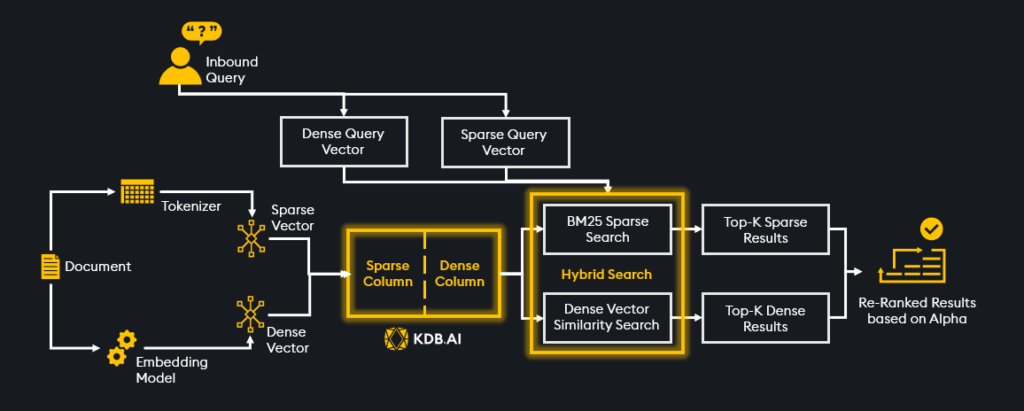
To learn hybrid search, we will use KDB.AI to identify relevant sparse and dense vectors related to an economic report on inflation. We will understand how to run dense, sparse, and combined hybrid search to increase the relevancy of our retrieved results.
Download the Jupyter notebooks at the GitHub repository.